How Machine Learning Is Revolutionizing Blockchain Analytics
Blockchain networks generate massive amounts of data every second – transactions, wallet activity, token movements, etc. Making sense of this data, spotting unusual patterns, and turning it into actionable insights is no small task. This is where machine learning (ML) transforms blockchain analytics into a powerful tool for developers, businesses, and regulators.
Machine learning is redefining how we interpret blockchain activity. From identifying fraudulent transactions to predicting market trends, it’s enabling faster, smarter decision-making across the decentralized ecosystem. Let’s explore how ML is changing the game in blockchain analytics and the companies leading this innovation.
Solving the Data Deluge With Machine Learning
Every blockchain transaction adds another layer to an already enormous pool of data. For example, Ethereum processes over a million transactions daily, each tied to complex metadata. Analyzing this manually? Practically impossible. ML excels at uncovering patterns, detecting anomalies, and automating these data-heavy processes.
ML-powered analytics dive deep into the data instead of just skimming the surface. Tools like Glassnode and Nansen use ML to monitor wallet behavior, highlight suspicious activity, and visualize on-chain trends. These tools help traders, businesses, and researchers track exactly what’s happening across networks.
Key Applications of Machine Learning in Blockchain Analytics
1. Detecting Fraud and Stopping Scams
Blockchain may be decentralized, but it’s not free from risks. Scams, hacks, and fraudulent schemes still plague the space. ML makes spotting these activities faster and more accurate.
Take Chainalysis, a leader in blockchain analysis. Their ML models sift through billions of transactions to detect illegal activities like ransomware payouts, phishing scams, or links to dark web marketplaces. When suspicious patterns emerge – like funds repeatedly flowing through high-risk wallets – Chainalysis flags them, providing law enforcement and businesses with actionable insights.
Another example is Elliptic, which uses ML to connect the dots between seemingly unrelated wallets. Analyzing historical transaction patterns helps exchanges and financial institutions identify accounts potentially involved in money laundering or other illicit activities.
2. Recognizing Transaction Patterns and Clusters
Not all blockchain wallets behave the same. Some are used for regular transactions, while others are hubs for whale trading, market manipulation, or automated activity. Like clustering and anomaly detection, ML algorithms group wallets based on behavior.
Covalent, for example, employs ML to map token flows across different chains. They identify patterns like which wallets interact frequently, whether transactions follow predictable cycles, or if any new behavior deviates from the norm. This insight can help developers monitor tokenomics or help users identify unusual activity that might indicate hacks or rug pulls.
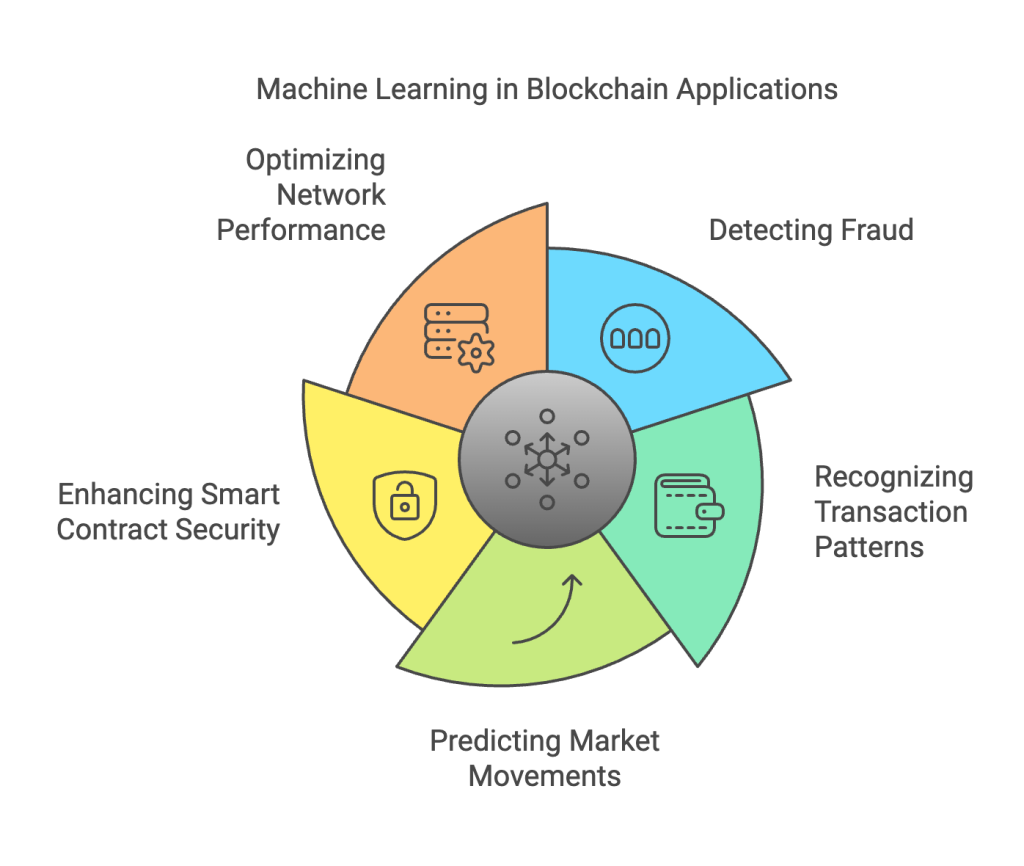
3. Predicting Cryptocurrency Market Movements
Data isn’t just on the blockchain in crypto – it’s everywhere. Price charts, social media chatter, trading volumes, and news cycles all influence markets. Machine learning brings this scattered data together and makes sense of it.
Platforms like Token Metrics combine blockchain activity with external data, such as social sentiment and trading trends. Their ML models help traders predict token prices, market momentum, and volatility. By combining on-chain analytics with sentiment analysis, they can forecast shifts in investor behavior before they happen.
Another example is Kaiko, which uses machine learning to analyze cryptocurrency trade data. By identifying patterns in exchange liquidity and order book trends, Kaiko provides market intelligence to institutional traders who want to optimize their strategies.
4. Enhancing Smart Contract Security
Smart contracts drive much Web3 innovation, from DeFi protocols like Uniswap to NFT marketplaces like OpenSea. However, bugs or vulnerabilities in these contracts can lead to devastating losses, as seen in exploits like the Poly Network hack.
This is where companies like Quantstamp use ML to audit smart contracts. Their algorithms detect risky code and potential vulnerabilities by learning from previous security breaches. Developers use these insights to patch flaws before deploying contracts, safeguarding user funds and project integrity.
5. Optimizing Blockchain Network Performance
Some blockchains, like Ethereum, struggle with network congestion and high gas fees. ML can analyze transaction behavior to identify bottlenecks and optimize performance. For example, reinforcement learning – a type of ML that trains systems to make better decisions over time – can recommend the most efficient ways to process transactions or manage network upgrades.
How Companies are Leveraging Machine Learning
1. Glassnode: Visualizing Blockchain Insights
Glassnode is known for its intuitive dashboards that show key blockchain metrics. They use ML to generate these insights, tracking everything from Bitcoin’s hash rate to Ethereum’s active addresses. This helps traders and developers quickly grasp network activity and make informed decisions.
2. Nansen: Connecting Wallets to Real Identities
Nansen takes ML one step further by labeling wallet activity with real-world identities. For instance, they might tag a wallet as belonging to a major trading platform like Binance or an NFT whale. This level of analysis helps users spot trends, such as large token movements, before a market shift.
3. Chainalysis and Elliptic: Keeping Crypto Clean
Both companies use ML to fight financial crimes. Chainalysis helps regulators and law enforcement track down stolen funds, while Elliptic focuses on giving businesses tools to ensure compliance with anti-money laundering (AML) regulations.
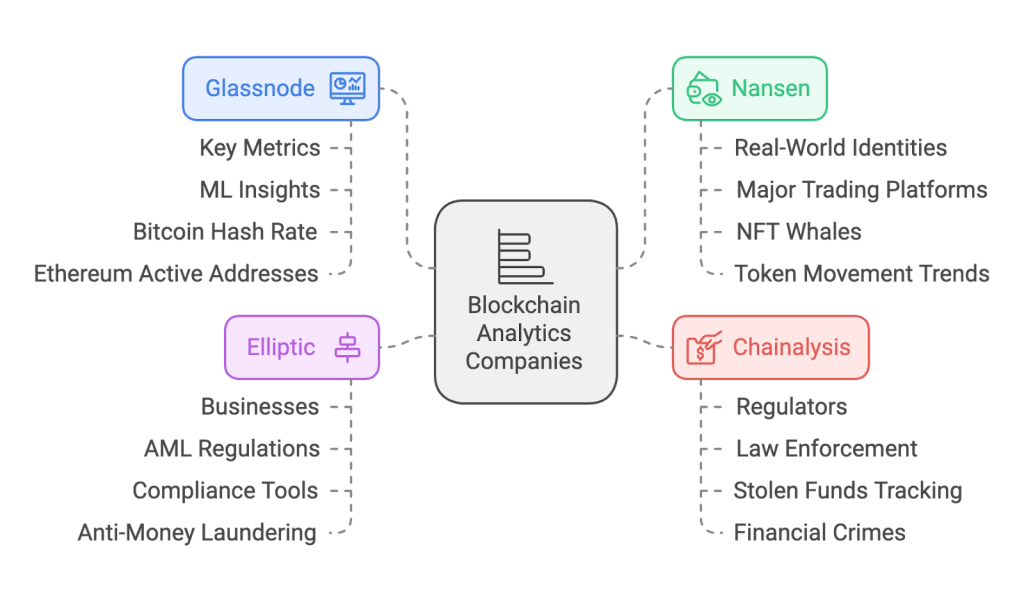
Challenges in Using Machine Learning for Blockchain Analytics
While ML offers immense potential, it’s not without challenges:
Conclusion
The synergy between blockchain and machine learning is still in its early days, but the potential is enormous. As blockchain adoption grows, so will the need for smarter tools to understand, secure, and optimize these networks. Companies like Chainalysis, Glassnode, and Quantstamp are already leading the charge, and new startups are entering the space daily.
Shortly, we can expect ML to play an even more significant role in:
- Real-time security monitoring: Instant detection of suspicious blockchain activity.
- Cross-chain analytics: Bridging insights across multiple blockchains for a complete market view.
- User-friendly tools: Simplifying analytics so everyone – not just developers – can benefit from blockchain insights.
Combining machine learning and blockchain analytics creates a smarter, safer, and more transparent ecosystem. Whether you’re a developer, trader, or blockchain enthusiast, this duo is reshaping how we interact with decentralized networks – and the possibilities are just getting started.